PMSoil: Predictive mapping of soil properties for the evaluation of soil functions at regional scale
Planning and decision-making for optimal use of soils requires spatial information on the potential of soils for particular soil functions. Soil function potentials are derived from soil basic properties by pedotransfer functions. However, detailed spatial soil property information in Switzerland is sparse. PMSoil is a broad collaborative effort to develop an applicable framework for digital soil property mapping and digital soil function assessment. Three study regions in Canton of Berne and Zurich were chosen to test the methodology.
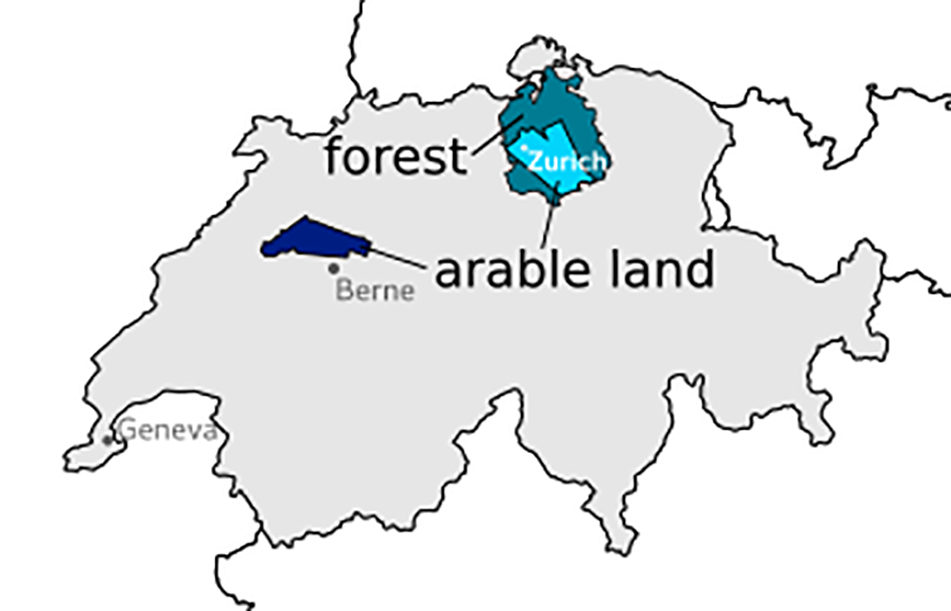
Figure 1: The three study areas: Forested area of the Canton of Zurich and the arable land within
the APEX flight campaigns to the south of Zurich and to the north of Berne.
For forest soils parameters describing soil acidification and for arable land water logging, nutrient cycling and agricultural production are of special interest. The developed soil property and function maps will be delivered to the soil agencies of the cantons and will hopefully help them to deal with the soil-related challenges they currently face. The tangible product of PMSoil will further serve as an important basis for the research of further two NRP68 projects: OPSOL, IMSoil.
The research of PMSoil is bundled into four packages:
- Workpackage A Harmonization of legacy soil data
- Workpackage B Terrain modelling and remote sensing for digital soil mapping
- Workpackage C Statistical modelling approaches for digital soil mapping (see below)
- Workpackage D Pedotransfer functions and soil function maps
Workpackage C: Statistical modelling for digital soil mapping
The STEP group has the lead for workpackage C. The focus is on advancement of statistical methodology and software development for digital soil property mapping. This includes the application of machine learning techniques, in particular the development of boosted structured regression models by component wise linear least squares as baselearners.
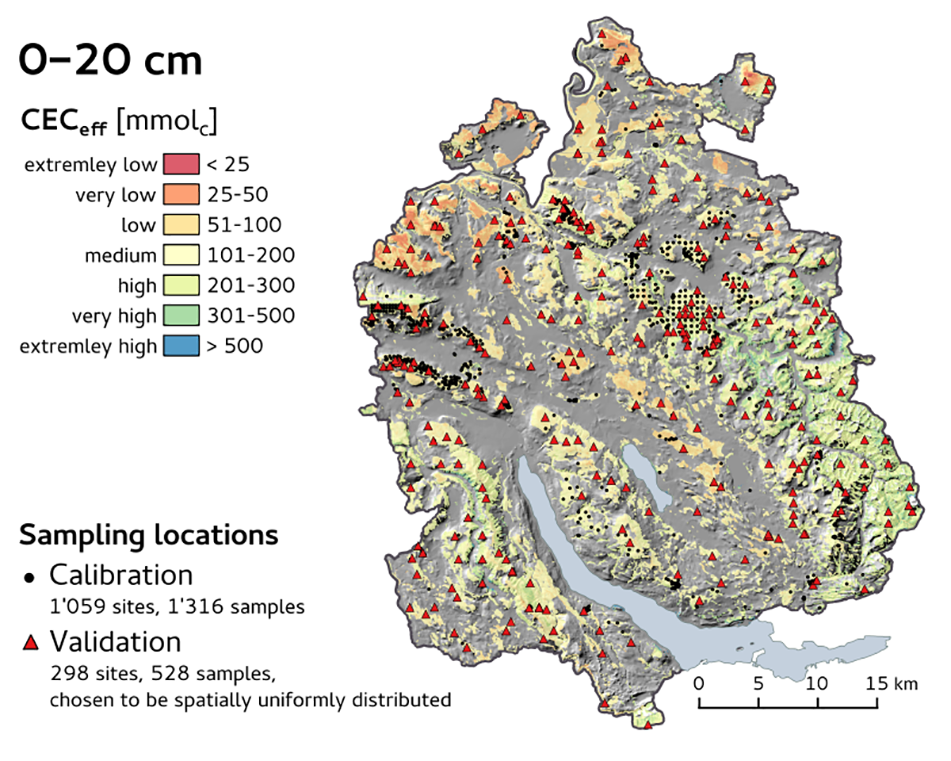
Figure 2: Map of the effective cation exchange capacity [mmolc] for the forested area of the Canton of Zurich.
According to first results for Zurich forests boosted structured regression efficiently selects model covariates from a large set. Due to the fully automatic model selection process simulation of the predictive accuracy will be possible. However, soil measurements are not readily available for subsoil layers. This will possibly restrict subsequent soil function evaluation.
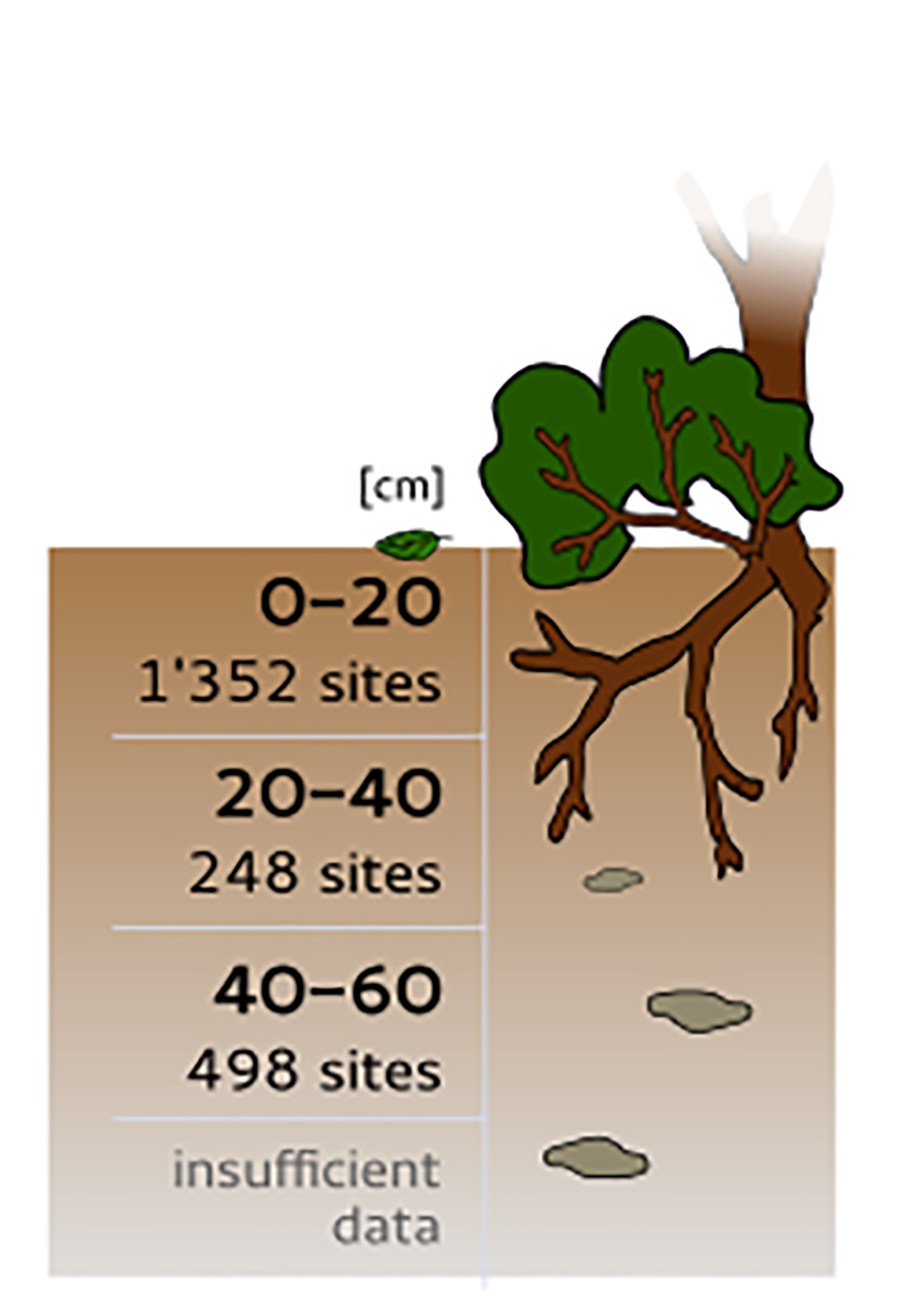
Figure 3: Soil data availability for effective cation exchange capacity measurements according to soil depth.
Team: Andreas Papritz (lead, STEP), Jiri Presler, Marco Carrizoni (Babu GmbH), Urs Zihlmann (Agroscope ART) and in collaboration with Martin Mächler (Seminar for Statistics, ETH), Madlene Nussbaum
Funding: Swiss National Science Foundation. National Research Programme NRP 68
Students: Madlene Nussbaum
Contact: andreas.papritz(at)env.ethz.ch